PARTICIPATING LABS
Bunne, Charlotte
Tailoring therapies to an individual’s unique molecular profile is essential for effective treatment, and this requires a deep understanding of the cellular and macromolecular mechanisms that influence treatment response. To achieve this, our lab develops novel artificial intelligence methods designed to mimic the structure and inner workings of biological systems, allowing them to be trained on large biomedical datasets from various experimental technologies. By leveraging these AI techniques inspired by biological systems, our work not only provides important contributions to personalized medicine but also impacts active machine learning research. As we unlock a deeper understanding of cellular mechanisms, we aim to enable more effective and individualized therapies for patients.
Personalized Treatments and Therapy Design
To advance personalized medicine, we need to design deep learning architectures that incorporate biomedical priors, scale to large biological datasets, and are deployable in the clinical setting. Such architectures will be essential for making reliable predictions of therapy outcomes and forecasting the molecular behavior of different drugs.
Our previous work has developed AI methods, such as diffusion and flow matching models as well as (vision) transformers, to learn and infer dynamic responses of single cells derived from cancer patients using both imaging and sequencing data. By integrating insights of the underlying biological mechanisms with concepts from dynamic systems theory, and contributing novel theoretical and algorithmic frameworks, these tools can forecast the dynamics of complex biological processes. Our efforts in that area directly translate into clinical applications: In a current observational clinical study of cancer patients within the Tumor Profiler consortium, our tools demonstrate the potential for meaningful treatment outcome predictions for a diverse set of patients.
Digital Diagnostics and the Vision of the Digital Twin
Building on insights from AI breakthroughs in vision and language and leveraging vast amounts of biological data, our current research aims to advance the vision of a virtual cell, a foundational AI-based model capable of predicting the behavior of healthy and diseased cells with broad applications for biomedical research and therapeutic development. AI virtual cells allow the creation of a patient’s medical digital twin, leading to faster diagnoses, more successful treatments, and the detection of rare diseases. Such machine learning models then allow simulating patient-specific treatment responses and providing an in silico environment for testing drug candidates and conduct diagnosis of diseases. Creating such virtual patient representations as well as digital diagnostics requires innovations on adaptive algorithms that navigate the constraints of clinical settings and the complexity of human biology.
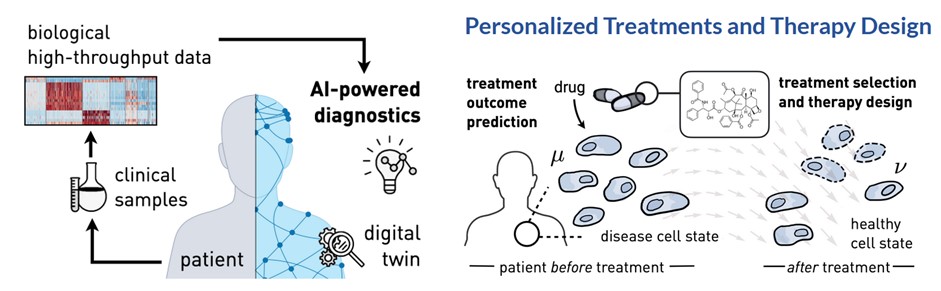
Key technologies
- (Generative) Artificial Intelligence
- Foundational Models
- Lab-in-the-Loop Technologies
Key biological questions
- Therapy and Drug Design
- Clinical Diagnostics
- Biomarker Discovery
Contact
EPFL BC BuildingChem. Alan Turing, 1015 Lausanne, Switzerland